MANTIS Ph.D. student José Pilartes-Congo recently defended his dissertation research proposal titled “Change Detection and Digital Twin Generation from Multi-Sensor and Multi-Scale 3D Data Fusion”.
——
His abstract read as follows:
Reality capture technologies incorporating 3D scanning and imaging techniques provide innovative and efficient means for measuring the geometric characteristics of built and natural environments. Common techniques include structure-from-motion / multi-view stereo (SfM/MVS) photogrammetry and lidar scanning, which provide dense and informative 3D point clouds, textured meshes, and digital elevation models (DEMs) that can be used to create digital records and repositories of geospatial data, thus supporting surveying and mapping efforts. These methods can be implemented on various remote sensing platforms such as uncrewed aircraft systems (UASs), traditional aircraft, or satellites to offer different extents of coverage, spatial resolution, and measurement accuracies. By facilitating accurate and effective monitoring of structural and environmental changes over time, these techniques can support a wide range of tasks such as project planning, asset management, and natural resource allocation. However, to effectively do so requires determining how to best exploit information captured in 3D data streams acquired from various remote sensing modalities, while addressing differences in data characteristics, measurement fidelity, and task suitability for different applications. This research explores the following question: How can reality capture (i.e., remote sensing) technologies providing 3D geospatial data at different perspectives, resolutions, geographical extents, and measurement fidelities be effectively and optimally integrated to support structural change detection and monitoring of built and natural environments? This research acknowledges that individual remote sensing modalities for acquiring 3D geospatial data have various advantages and limitations and examines ways to optimally fuse different datasets in a way that one complements the other, resulting in more informative and useful 3D geospatial datasets for change detection applications. With this consideration, this research explores data acquired from ground, air, and space, using photogrammetry and lidar scanning technologies, as well as associated algorithmic and processing techniques for data calibration, georeferencing, accuracy assessment, and data fusion for generating 3D digital twins of the environment. Ultimately, the research seeks to develop a digital twin framework able to ingest multi-sensor, multi-scale 3D data at different spatial resolutions and temporal frequencies, for geospatial change detection analyses to support surveying and monitoring activities, especially for transportation roadway corridors (built environments) and dynamic coastlines (natural environments).

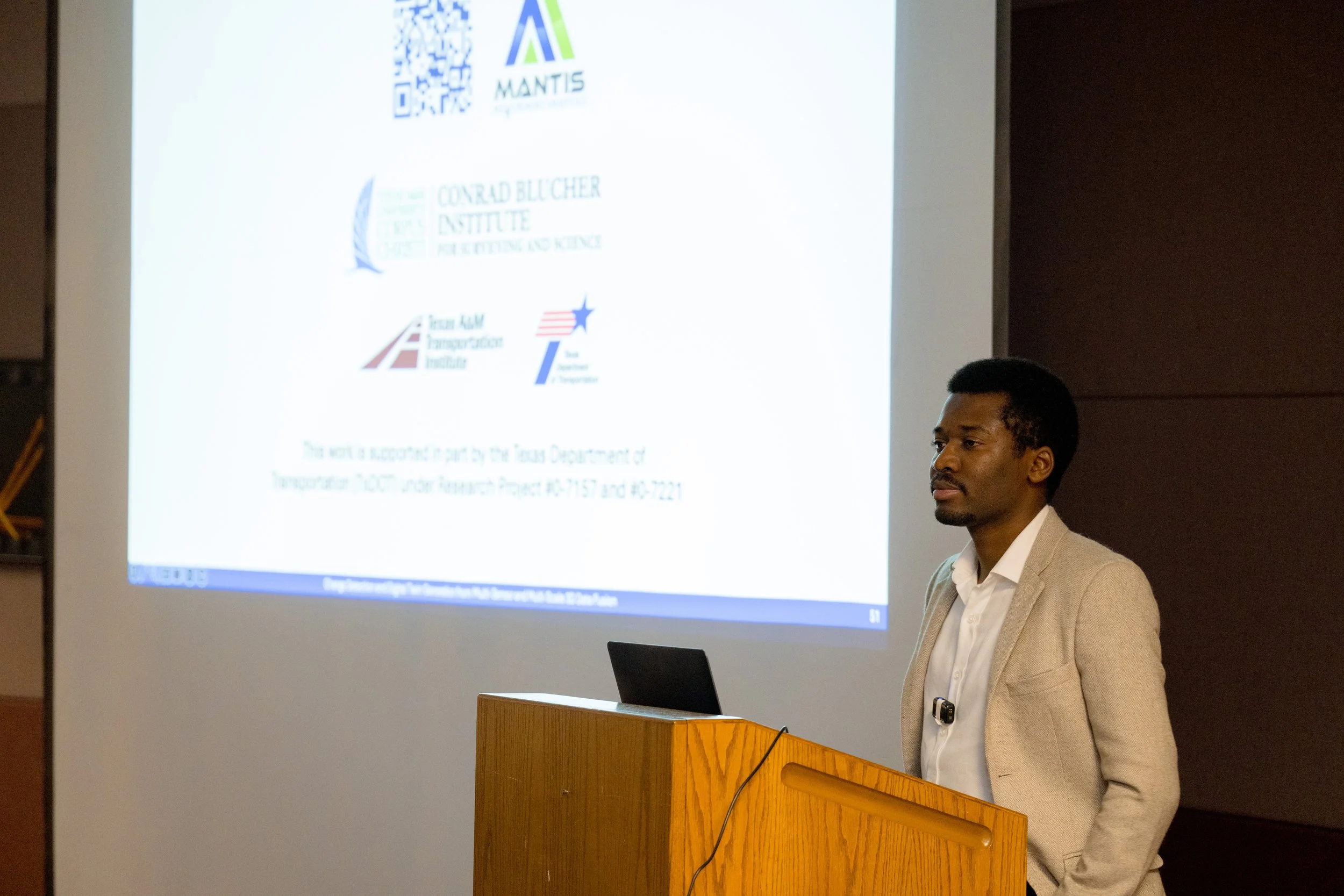


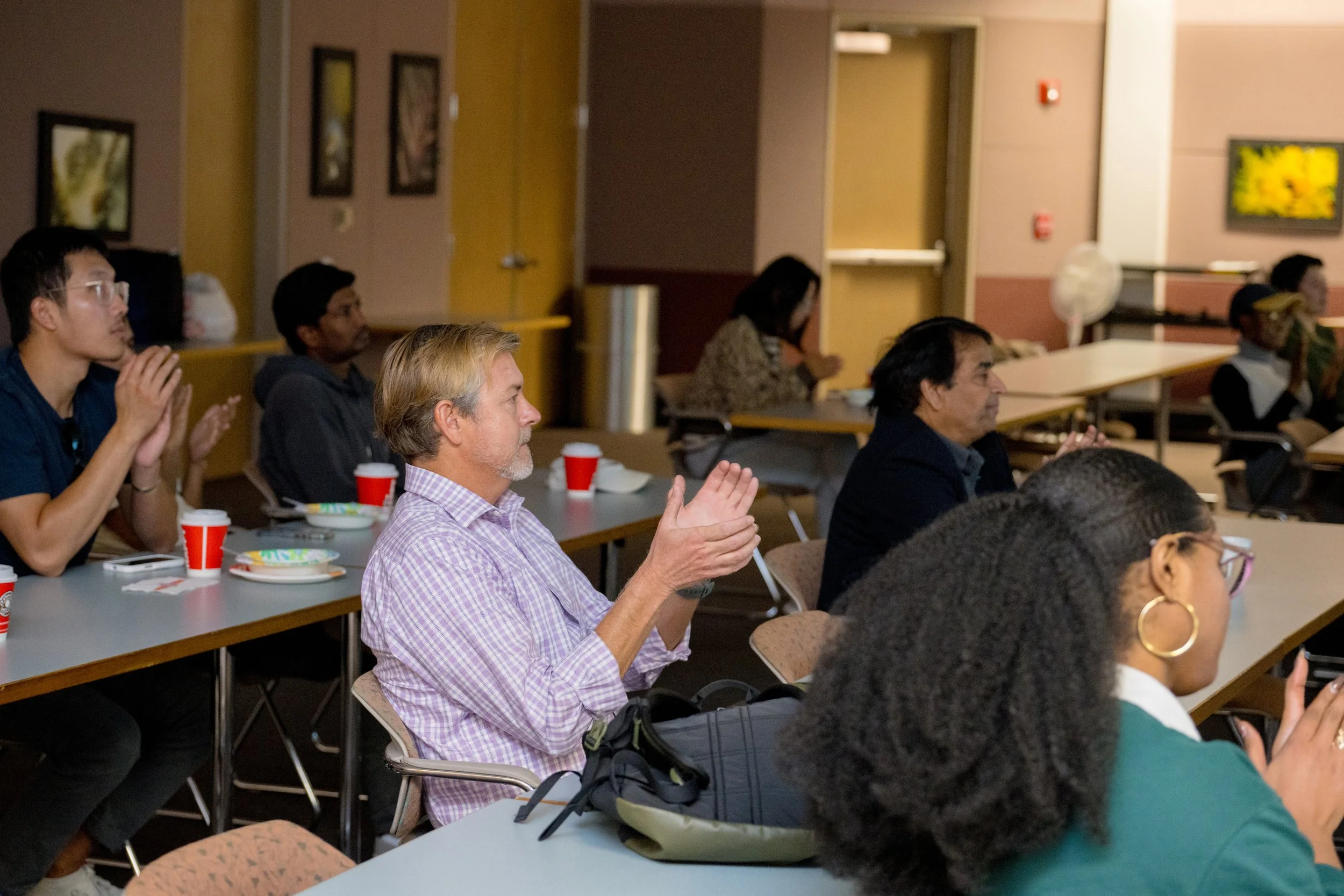


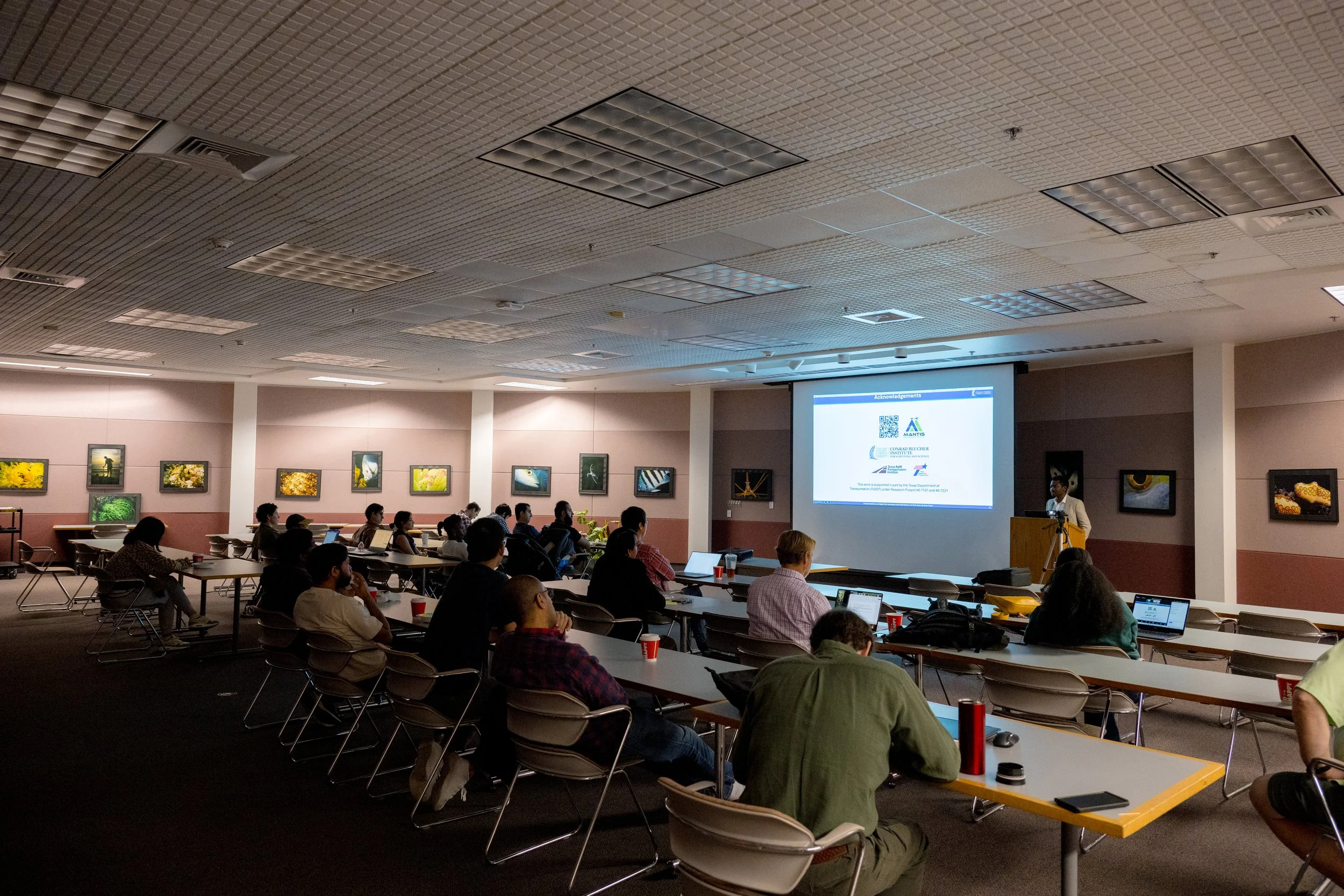